IIM Calcutta
Implementing Timetable Scheduling at IIM Calcutta Using Automation with Demand Estimation Data, Course Clash Matrix, and Faculty Preferences
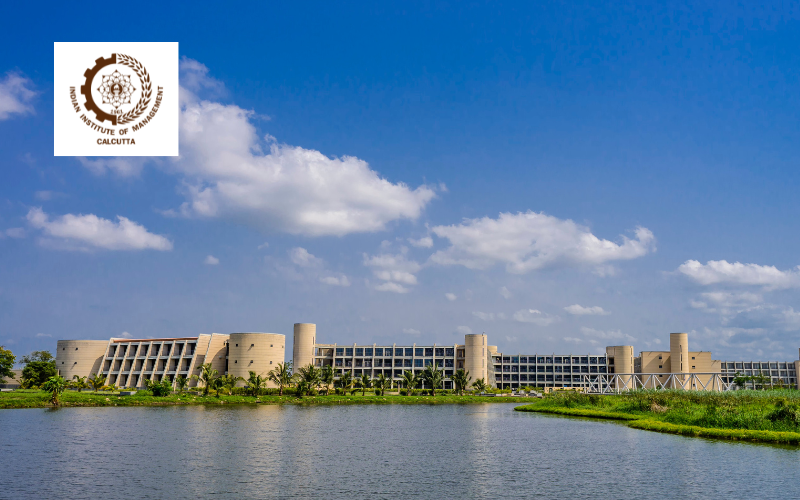
Overview
IIM Calcutta faced challenges in creating effective timetables each academic term. The manual process of scheduling classes, taking into account demand for courses, avoiding scheduling conflicts, and considering faculty preferences, had become time-consuming and error-prone. The school sought to streamline this process through automation, using data-driven methods to improve efficiency and accuracy. This case study outlines how the school implemented an automated timetable scheduling system based on demand estimation data, a course clash matrix, and faculty preferences, significantly improving the process.
The Problem
Before automation, the school’s scheduling team manually assigned courses to available time slots, trying to balance several variables:
- Demand Estimation Data: Predicting student enrollment for various courses.
- Course Clash Matrix: Avoiding scheduling conflicts between courses often taken by the same students.
- Faculty Preferences: Accommodating instructors' preferred teaching times and avoiding overbooking them.
This process involved back-and-forth communication between faculty, the administration, and students. As the number of courses and faculty increased, the scheduling process became more complex, often resulting in inefficiencies, scheduling conflicts, and under-utilization of classrooms. There was also a growing dissatisfaction among students who could not enroll in their preferred classes due to poor time slot allocation.
Objectives
The primary goals of the automation initiative were:
- Increased efficiency: Automating the scheduling process to save time.
- Better demand matching: Using enrollment data to ensure popular courses were scheduled in larger venues and at optimal times.
- Conflict resolution: Preventing course clashes and double bookings for both students and faculty.
- Faculty satisfaction: Incorporating faculty preferences without manual intervention.
- Flexibility: Allowing quick adjustments in response to changes in student enrollment or faculty availability.
Solution: An Automated Timetable Scheduling System
The business school implemented a timetable scheduling system built around three key data sources: demand estimation data, a course clash matrix, and faculty preferences. The system also leveraged optimization algorithms to create conflict-free schedules.
1. Demand Estimation Data
The school had historical data on student enrollment patterns and trends for each course, which was fed into a demand estimation model. This model predicted the number of students likely to enroll in each course, enabling the system to assign appropriate time slots and classroom sizes. Popular courses were given priority for larger venues and time slots with high student availability.
2. Course Clash Matrix
A matrix was developed that mapped out common course pairings that students often take together. The matrix identified potential clashes if two or more courses were scheduled simultaneously. The automated system used this data to ensure that no two courses likely to be taken by the same students were scheduled at the same time.
3. Faculty Preferences
Faculty members were asked to submit their preferences for teaching times (e.g., mornings, afternoons) and any constraints (e.g., research commitments, personal scheduling conflicts). These preferences were then incorporated into the scheduling system. The system aimed to accommodate as many preferences as possible while still ensuring a balanced and efficient schedule.
4. Optimization Algorithm
The system used a constraint-based optimization algorithm, designed to find the best possible schedule based on the available data. The algorithm balanced several competing priorities:
- Minimizing course clashes for students.
- Assigning courses to suitable classrooms based on demand predictions.
- Accommodating faculty preferences.
- Ensuring balanced use of classrooms and time slots to avoid overloading any one period.
Implementation
The implementation process involved several phases:
- Data Collection and Integration: Historical enrollment data, course relationship data (clash matrix), and faculty preferences were gathered and standardized for integration into the new system.
- System Development: The timetable scheduling software was customized for the school’s needs, with functionalities added to input, process, and output schedules based on the three key data sets.
- Testing and Validation: A series of test schedules were generated and validated against historical outcomes. Faculty and administrative staff reviewed the test schedules to ensure the system was meeting their needs.
- Training and Rollout: Training sessions were held for administrative staff on how to input data, review schedules, and make minor manual adjustments when needed.
Results
The new system led to several immediate improvements:
- Increased Efficiency: The time spent creating timetables was reduced by more than 70%. What used to take weeks was now done in a matter of days.
- Fewer Scheduling Conflicts: There was a marked reduction in student complaints about course clashes, with the system resolving over 95% of potential conflicts identified by the course clash matrix.
- Optimized Classroom Use: Classrooms were better utilized, with larger classes assigned to appropriately sized rooms based on demand estimation.
- Faculty Satisfaction: Over 85% of faculty preferences were successfully incorporated into the final schedule, improving satisfaction among instructors.
- Improved Flexibility: The system allowed for quick re-scheduling in response to changes in student enrollment or unexpected faculty absences, without needing to start from scratch.
Challenges and Lessons Learned
While the automated scheduling system proved successful, several challenges were encountered:
- Data Quality: The system’s effectiveness depended heavily on accurate demand estimation and up-to-date faculty preferences. Initial data inconsistencies required additional cleaning and verification.
- Faculty Buy-In: Some faculty members were initially skeptical of the new system, fearing it would reduce their flexibility. Clear communication and the system’s ability to accommodate most preferences helped ease concerns.
- Customization: The business school required a custom solution to fit its specific needs, which increased initial development time and costs. However, the long-term benefits of efficiency gains outweighed these initial hurdles.
Conclusion
By implementing an automated timetable scheduling system that leveraged demand estimation data, a course clash matrix, and faculty preferences, the business school significantly improved its scheduling process. The system not only saved time and reduced errors but also improved faculty and student satisfaction by creating more balanced, conflict-free timetables. This case demonstrates the value of data-driven decision-making and automation in addressing complex administrative challenges in higher education.
More Case Studies
Visualise University Automation
Discuss about your Operational Process Pain-points
Discover the Gaps and apply Targeted Solutions
Experience Automation powered Student Success with 10X Impact